Editor’s Note: Chennai Meetup Wrap-Up & Preparation work started for DEWCon
I am so grateful for the enthusiastic participants who made our Chennai Data Heroes- Community for Data Folks meetup vibrant! Big thanks to our insightful speakers,
Pradheep Arjunan - Shared insights on AZ's journey from on-prem to the cloud data warehouses.
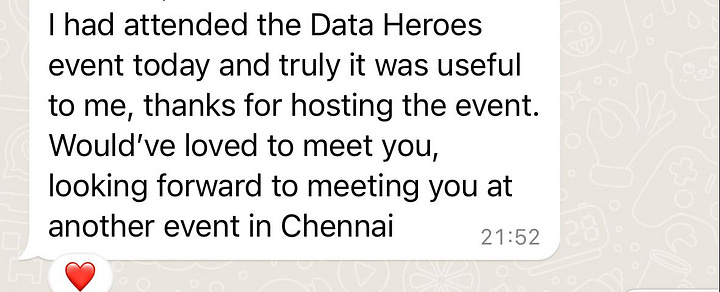
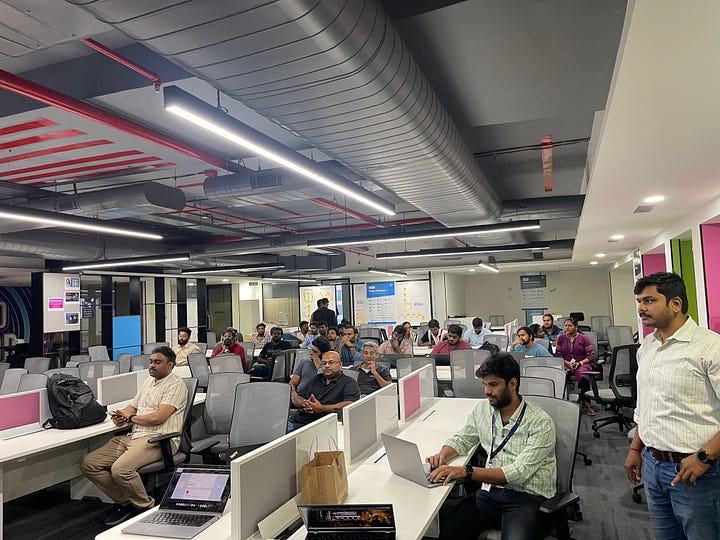
Thanks to Ideas2IT Technologies for hosting us in their fantastic space.
Google: Croissant- a metadata format for ML-ready datasets
Google Research introduced Croissant, a new metadata format designed to make datasets ML-ready by standardizing the format, facilitating easier use in machine learning projects. Croissant builds upon existing standards like schema.org, enhancing them with layers specific to ML needs, including data organization and ML semantics. Major ML dataset repositories and frameworks support Croissant, simplifying the discovery, preparation, and utilization of datasets for machine learning practitioners by standardizing and organizing them.
https://blog.research.google/2024/03/croissant-metadata-format-for-ml-ready.html
Piethein Strengholt: Data Quality within Lakehouses
This article emphasizes the importance of data quality in data lakehouses, outlining the consequences of neglecting it and advocating for a structured approach through a governance framework. It distinguishes between intrusive and non-intrusive data quality management, advocating for clear governance to address quality issues. Furthermore, it presents a common lakehouse design, emphasizing data validation across bronze, silver, and gold layers, and suggests technologies and practices for maintaining high data quality, emphasizing continuous improvement and the critical role of standardization.
https://piethein.medium.com/data-quality-within-lakehouses-0c9417ce0487
NVIDIA: What Is Trustworthy AI?
NVIDIA's blog post on Trustworthy AI defines it as an AI development approach prioritizing safety and transparency, emphasizing the importance of developing AI systems that people can trust. The post outlines key principles such as privacy, safety, security, transparency, and non-discrimination, highlighting NVIDIA's initiatives like federated learning, guardrails for AI applications, and transparency tools.
https://blogs.nvidia.com/blog/what-is-trustworthy-ai/
Sponsored: Streamlining Data Analytics with a Semantic Layer - How Ramsoft uses Cube, AI & Microsoft Fabric to ensure customers have customized and intuitive embedded analytics
Come join Cube’s webinar on March 13 exploring how Ramsoft uses a universal semantic layer to improve the embedded analytics experience for external users.
Hear directly from Dhyan Shah, Ramsoft’s Product Manager, as he determines factors for his technology choices that help him navigate working with LLMs, and how this experience will drive his future projects.
Date: Wednesday, March 13, 2024 at 9 am PT | 12 pm ET
Featured Speakers:
Jen Grant, COO of Cube
Dhyan Shah, Product Manager of Ramsoft
https://bit.ly/cube-ramsoft-webinar
StackOverFlow: Best practices for building LLMs
Intuit is advancing its product development by creating custom in-house large language models (LLMs) to bridge the gap between off-the-shelf capabilities and specific customer needs. This culminated in the creation of GenOS, an operating system for developing GenAI-powered features. Intuit advocate starting with existing models and enhancing them through prompt engineering or training on domain-specific knowledge before considering custom LLM development. This approach, coupled with balancing model size against resource costs and continuously updating models to maintain accuracy, underlines a principled strategy for integrating GenAI into products and engineering workflows.
https://stackoverflow.blog/2024/02/07/best-practices-for-building-llms/
Intuit: Building a flexible platform for optimal use of LLMs
Intuit has developed a GenAI operating system, GenOS, to harness the power of generative AI and large language models (LLMs) for various financial services. This platform enables Intuit developers to access, compare, and customize various LLMs, including proprietary ones tailored for financial challenges, aiming to enhance productivity and create personalized experiences for their vast customer base. Addressing challenges such as maintaining a dynamic LLM catalog, matching models to specific use cases, fine-tuning for optimization, and promoting responsible development, Intuit's approach underscores the complexity and potential of deploying GenAI in financial technologies.
Netflix: Evolving from Rule-based Classifier - Machine Learning Powered Auto Remediation in Netflix Data Platform
Netflix has initiated a project to integrate Machine Learning (ML) with its existing rule-based classifier for operational automation in big data job management, focusing on auto-remediation. The system handles specific Spark job errors and significantly improves efficiency by automating the correction of memory configuration errors and other unclassified errors, demonstrating a notable reduction in error rates and operational costs.
Microsoft: Evaluating Large Language Model (LLM) systems: Metrics, challenges, and best practices
The Microsoft article outlines strategies for evaluating Large Language Model (LLM) systems, highlighting the distinction between evaluating standalone LLMs and LLM-based systems. It emphasizes the iterative nature of evaluation, integrating continuous integration, evaluation, and deployment (CI/CE/CD) within LLMOps for managing LLM applications. The piece discusses the importance of tailoring evaluation metrics to specific use cases, the challenges of creating golden datasets for accurate evaluation, and the balance between offline and online evaluations for comprehensive assessment. It also explores the use of AI in generating and evaluating datasets, advocating for Responsible AI metrics to ensure ethical use.
Yelp: Building data abstractions with streaming at Yelp
Yelp has revamped its data pipeline to stream huge volumes of data in real time, focusing on building robust data abstractions for offline and streaming data consumers. Using a unified stream for all relevant business property data, Yelp ensures a consistent and user-friendly format for data consumers, eliminating the need to navigate between different business attributes and features. This approach enhances data discovery, consumption, and ease of use while addressing challenges such as weak encapsulation and maintenance difficulties in the previous architecture.
https://engineeringblog.yelp.com/2024/03/building-data-abstractions-with-streaming-at-yelp.html
Richard Crowley: SOC 2 compliance for startups and first-timers
While SOC 2 might seem outside a data engineer's wheelhouse, understanding its principles is key. Data engineers build the systems that store and process sensitive information. By following SOC 2 guidelines, they can implement strong authentication, encryption, and data handling practices. The author writes an excellent three-part series that introduces SOC2 compliance.
https://blog.substrate.tools/soc2-part1/
https://blog.substrate.tools/soc2-part2/
https://blog.substrate.tools/soc-2-compliance-for-startups-and-first-timers-part-3/
All rights reserved ProtoGrowth Inc, India. I have provided links for informational purposes and do not suggest endorsement. All views expressed in this newsletter are my own and do not represent current, former, or future employer” opinions.